Becoming an AI-ready Designer: Research & Analysis (Pt. 2)
Methods, tools and mindset to incorporate in your day-to-day
Hey everyone, Filippos here 👋. I originally started writing this as one post but it became so big that I decided to split into multiple parts. I had an incredible time writing this series of posts and hopefully it will be as insightful to you as it has been for me.
If you are new to product design, I also recommend checking out my product design masterclass, a self-paced, end-to-end product design course for beginners and mid-level designers.
Prologue
This is the second part of a series of posts on becoming AI-ready as a Product Designer. The series explores tools, workflows and methods that, as product designers, we should familiarize ourselves with, so that we are ready for a new AI-powered era in product design and development.
2: Research & Analysis (this post)
3: Building Products & Prototypes
4: Wireframing & Design
5: Mindset
Let’s dive into it.
Desk research
(Method: Summarising, Analyzing)
I’m a huge advocate of using AI for desk research (secondary research). It’s one of the clearest, biggest optimizations in a workflow I’ve experienced so far, and it can empower more people in being comfortable doing their own desk research.
Depending on your type of research, I’d recommend the free version of Perplexity for most day-to-day work, and a specialized model such as Gemini with Deep Research (paid) if you are tackling something complex.
Day-to-day research
Example use case: You are trying to understand how people behave around a certain topic e.g. mobile banking. Instead of using a search engine, go into Perplexity and ask a number of questions to understand a topic.
Perplexity works similarly to older generation search engines, but does a great job:
Summarizing the results
Suggesting follow-ups (one of my favorite features)
Showing citations very clearly, allowing you to dive into the actual data
In this example, I started with a wide search around mobile banking apps in the UK.
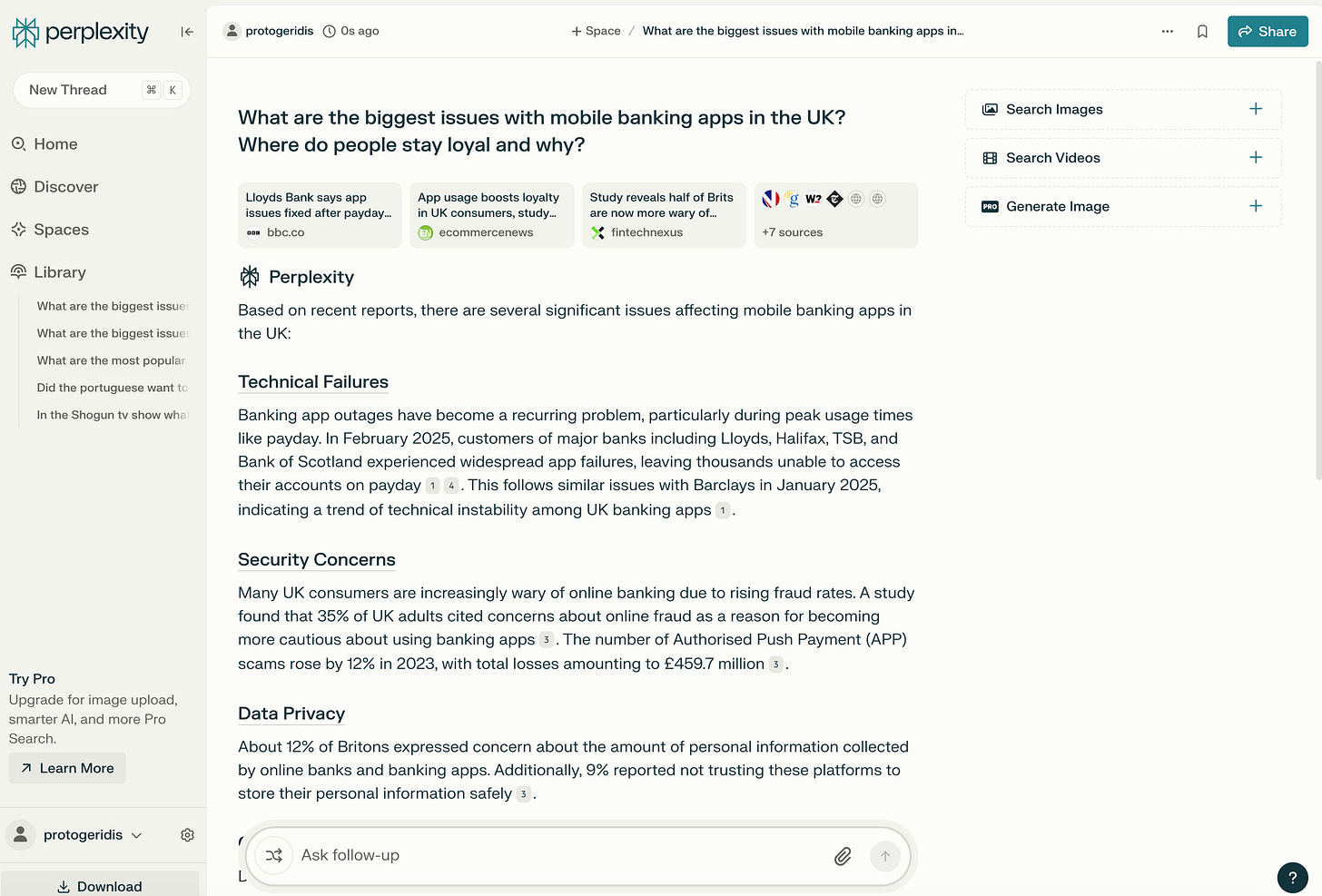
Because most of the sources were big publisher articles, I then narrowed down the search by asking Perplexity to only fetch results from Reddit and other communities, as I wanted to see how consumers actually think and talk about the topic.
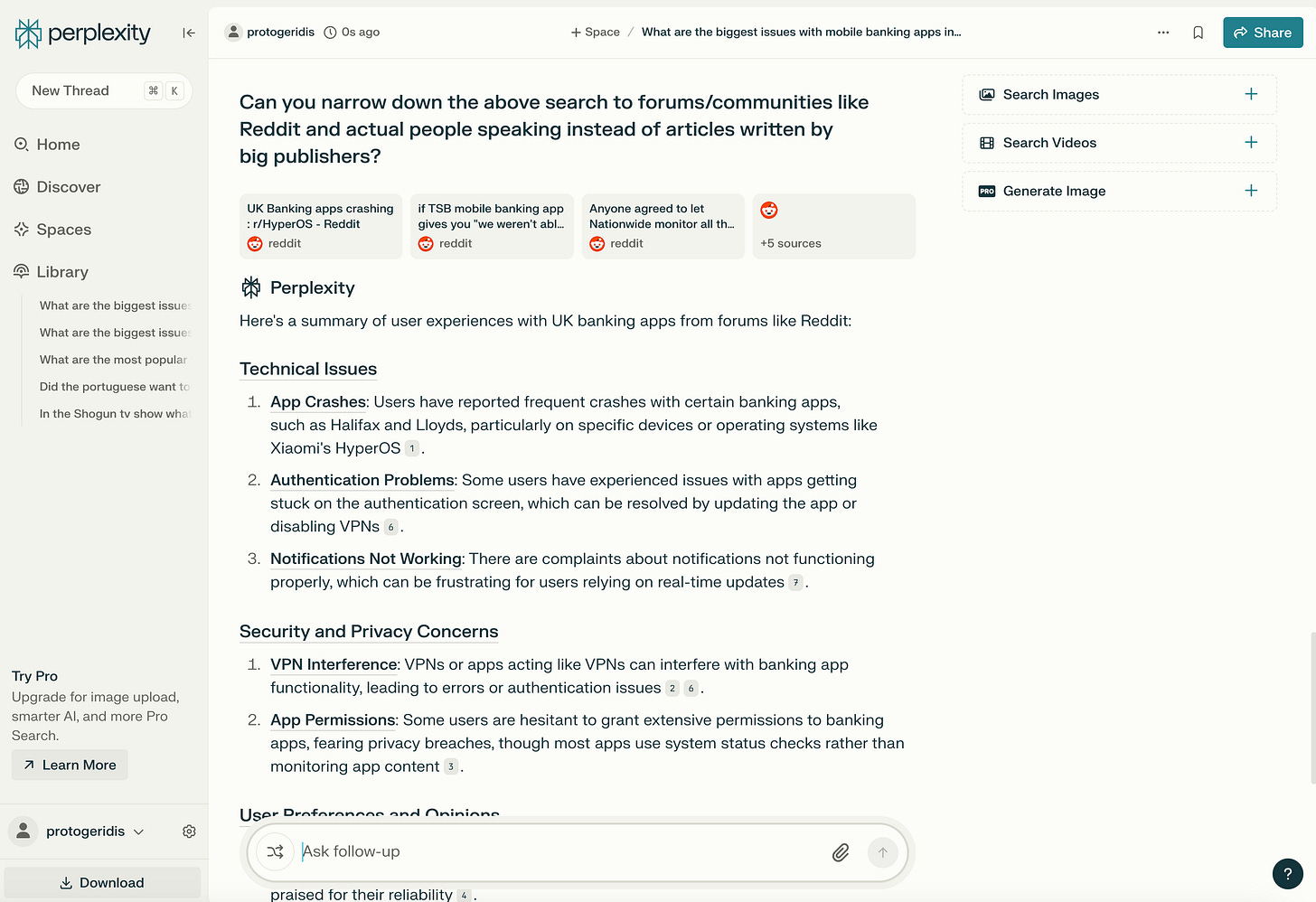
Deep research
Example use case: You are a founding designer, preparing for the launch of a new product in a market (e.g. form builders) and trying to better understand it. In this case I’d sign up for Google Gemini Advanced and use its Deep Research Model to do a thorough market analysis.
I started by giving context on what I was trying to do:
🤖 Prompt: I want to run some competitor research on form-building SaaS products e.g. Typeform. Please find the biggest players in the market and outline:
- Their pricing
- Their target market e.g. startup vs. enterprise
- Key customers using them
- Feature breakdown along with how users value them
- What users like about them vs. complain about
- What you think the biggest opportunities are if we were to create a new startup in the space today.
The way that Deep Research models work is by creating an action plan, verifying it with the user, and then doing extensive analysis on multiple sources before summarizing all results in a separate document.
It genuinely feels like having a mini consulting firm working alongside you.
Verdict: Extremely powerful 👏
Skill to learn: Research prompting, follow-up prompts to add specificity.
Transcribing research
This section will be no news to anyone and therefore quite short, as transcription was one of the first workflows that saw a huge influx of new tools delivering a high quality of results.
Tl;dr, transcribing research sessions is extremely effective using AI. You should be using a tool to transcribe and summarize all research sessions. Just pick one that fits your team’s needs and budget.
You can pick from a wide range of tools:
Gemini for Google Meet
Granola (Mac only)
Tldv.io (useful if you also want video highlights and aren’t using a specialized research tool)
To more specialised research tools such as:
Most of these tools offer free/trial versions, with their pricing starting from $15-20/user per month, so feel free to explore and pick a tool that you can afford and your team is happy with.
Verdict: Extremely powerful 👏
Skill to learn: None, they are all automated.
Analyzing user research
(Method: Summarising, Analyzing)
Via transcripts
This is one of my favorite workflows.
If you are using research transcripts to analyze research (as per the section above), one of the simplest yet most effective methods of analyzing is to feed your transcripts into ChatGPT or Claude and ask it to analyze them.
Example use case: You are a product designer working on a new healthtech product that helps people with digestive issues. You have run a series of discovery interviews to identify what the biggest problems and opportunities are, but are very short on time to manually analyze everything.
🤖 Prompt: You are a leading UX Researcher. Please review this transcript to identify relevant exceprts that address the research question [question]. Generate phrases (codes) that best represent the excerpts identified. Each code should be between two to five words.
Present your results in a table format. One column for the code, and one column for the participant’s quote(s).
(In the screenshots below I’m using a sample interview transcript taken from The Coding Manual for Qualitative Researchers by Johnny Saldaña)
ChatGPT did a really great job identifying quotes and coding them. It did miss some information that I thought was important though, which is why when using AI for research, it’s paramount that you double check the results.
To tackle this, one thing I have found quite useful is asking the chat to review the transcript again and add any additional quotes for each code.
Once I was happy with the notes form my first transcript, I asked ChatGPT to review additional transcripts and expand the table to account for multiple participants.
Tip: As with before, experiment with both ChatGPT and Claude to see which one creates a more accurate analysis and pick based on your preferences.
Tip: One headache that you will encounter is that — in their free plans — both chats have fairly small text length limits. A plugin I have found useful to tackle this is Split Long Text for ChatGPT, which splits your text into multiple parts and creates a prompt for the chat to wait for all the parts before doing the analysis. It’s not perfect, but it works.
Verdict: Very powerful, with a few headaches ⚡
Skill to learn: Summarizing, analyzing data through AI.
Via whiteboarding tools
If you are taking research notes in a whiteboarding tool, you can try using Miro and Figjam’s AI summary and clustering features. They are far from perfect, but can quickly help create some high level summaries of sticky notes that you can use as a reference point before diving in on your own.
The big caveat here is that visual note taking (via sticky notes) doesn’t fully encapsulate what the participant is saying or what the question is, and therefore might miss a lot of important context. I have found that depending on the quality of the notes, results here vary significantly, and therefore I can’t recommend this as a reliable way to analyze data.
In summary, using AI tools to analyze or summarize information captured in sticky notes isn’t very effective, purely because of the nature of whiteboarding tools which are meant to be scrappier.
I’d rather recommend you use an AI-powered transcript along with your sticky notes to accurately analyze a piece of research. Use the sticky notes as a reference point of what’s important, but then cross-reference with a transcript to get the highest level of accuracy.
Verdict: Highly dependent on how you note-take. Powerful when notes are very accurate, but not that useful when notes don’t encapsulate the full context or intention behind the note, which is usually the case.
Skill to learn: Summarizing, analyzing data through AI.
Hope you enjoyed this post in the series, I had a blast writing it. If you’d like to see more in-depth content on any of the above areas, I’d love to know in the comments. As always, if you found this insightful, consider restacking ♻️.
If you are new to product design, I also recommend checking out my product design masterclass, a self-paced, end-to-end product design course for beginners and mid-level designers.
Highlight of the month ✨
Fount is a wonderful website that gathers curated resources for designers. I’ve always kept personal notes of interesting resources, tools, tutorials but it has become a huge mess that’s impossible for me to manage, which is why I’ve been using Fountn more recently to find and bookmark resources until I have time to visit them. Hope you find it useful!
Wow, really interesting! Thank you!!!
Looking forward to seeing another one!